How to Overcome Data Quality Challenges in Informatics Projects
Informatics Magazine

How to Overcome Data Quality Challenges in Informatics Projects
Efficient informatics projects hinge on the quality of data used; yet, addressing this pivotal aspect remains a formidable challenge for many organizations. This article explores practical strategies to preempt and resolve data quality issues, emphasizing the integration of automated tools, solid data governance, and proactive collaboration between technical and business teams. By adopting these approaches, businesses can ensure data accuracy from the outset, reducing costs and improving decision-making.
- Address Data Quality Issues Early
- Use Automated Data Quality Tools
- Establish Clear Data Governance Policies
- Implement Data Profiling and Monitoring
- Ensure Data Accuracy at the Source
- Foster Collaboration Between IT and Business
Address Data Quality Issues Early
During a critical project involving a healthcare client's electronic health record system, we encountered a major data quality issue. Patient records contained duplicate entries and incomplete fields, which risked disrupting care continuity and compliance. Our team discovered this when integrating new data analytics tools, as inconsistent inputs created errors in reporting. It was clear that these discrepancies had to be addressed before proceeding further.
We tackled the issue by first identifying patterns in the errors using automated data validation tools. Once we had a clearer picture, our team manually reviewed the most critical datasets and collaborated closely with the client's staff to fill in missing information. To prevent future problems, we implemented a streamlined process for entering and validating data at the source. We also trained their staff on maintaining accurate records, ensuring long-term improvements.
This experience taught me the importance of early detection and communication. Addressing data quality issues requires both technical solutions and human intervention. Clear collaboration between teams can resolve immediate problems and foster habits that improve data reliability. It reinforced my belief that solving data challenges is just as much about people as it is about technology.
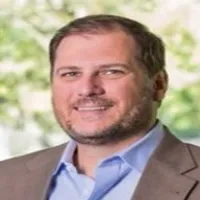
Use Automated Data Quality Tools
Automated data quality tools can significantly improve the consistency and accuracy of your data. These tools help identify and correct errors, reducing the time and effort spent on manual data cleaning. They can also support continuous monitoring, ensuring that data quality issues are detected and addressed promptly.
Utilizing these tools will lead to more reliable data for decision-making. Embrace the efficiency of technology to keep your data precise and dependable.
Establish Clear Data Governance Policies
Establishing clear data governance policies is essential for maintaining high-quality data. These policies define roles, responsibilities, and processes for data management, ensuring that everyone follows the same standards. A well-governed data environment helps prevent inconsistencies and inaccuracies.
It also provides a framework for handling data issues as they arise. Take action now to create and enforce robust data governance policies.
Implement Data Profiling and Monitoring
Implementing data profiling and monitoring techniques can help overcome data quality challenges in informatics projects. Data profiling involves analyzing data to get insights into its structure, content, and quality, which helps in identifying potential issues. Regular monitoring can detect and rectify data quality problems before they escalate.
This proactive approach ensures that data remains accurate and reliable over time. Start leveraging data profiling and monitoring today to maintain high data standards.
Ensure Data Accuracy at the Source
Ensuring data accuracy at the source is vital for preventing data quality issues later in the process. This means implementing checks and validation mechanisms where the data is initially generated or collected. By catching errors early, you can reduce the amount of bad data entering your systems.
This leads to more accurate analytics and better decision-making. Commit to enhancing data accuracy from the start.
Foster Collaboration Between IT and Business
Fostering collaboration between IT and business teams can significantly improve data quality in informatics projects. When these groups work together, they can better understand each other’s needs and challenges. IT professionals can create systems that meet business requirements, while business teams can provide valuable insights into the data.
This cooperation leads to more effective data management practices. Encourage your IT and business teams to collaborate more closely.